-
Notifications
You must be signed in to change notification settings - Fork 0
/
Copy pathBayes.Rmd
524 lines (353 loc) · 13 KB
/
Bayes.Rmd
1
2
3
4
5
6
7
8
9
10
11
12
13
14
15
16
17
18
19
20
21
22
23
24
25
26
27
28
29
30
31
32
33
34
35
36
37
38
39
40
41
42
43
44
45
46
47
48
49
50
51
52
53
54
55
56
57
58
59
60
61
62
63
64
65
66
67
68
69
70
71
72
73
74
75
76
77
78
79
80
81
82
83
84
85
86
87
88
89
90
91
92
93
94
95
96
97
98
99
100
101
102
103
104
105
106
107
108
109
110
111
112
113
114
115
116
117
118
119
120
121
122
123
124
125
126
127
128
129
130
131
132
133
134
135
136
137
138
139
140
141
142
143
144
145
146
147
148
149
150
151
152
153
154
155
156
157
158
159
160
161
162
163
164
165
166
167
168
169
170
171
172
173
174
175
176
177
178
179
180
181
182
183
184
185
186
187
188
189
190
191
192
193
194
195
196
197
198
199
200
201
202
203
204
205
206
207
208
209
210
211
212
213
214
215
216
217
218
219
220
221
222
223
224
225
226
227
228
229
230
231
232
233
234
235
236
237
238
239
240
241
242
243
244
245
246
247
248
249
250
251
252
253
254
255
256
257
258
259
260
261
262
263
264
265
266
267
268
269
270
271
272
273
274
275
276
277
278
279
280
281
282
283
284
285
286
287
288
289
290
291
292
293
294
295
296
297
298
299
300
301
302
303
304
305
306
307
308
309
310
311
312
313
314
315
316
317
318
319
320
321
322
323
324
325
326
327
328
329
330
331
332
333
334
335
336
337
338
339
340
341
342
343
344
345
346
347
348
349
350
351
352
353
354
355
356
357
358
359
360
361
362
363
364
365
366
367
368
369
370
371
372
373
374
375
376
377
378
379
380
381
382
383
384
385
386
387
388
389
390
391
392
393
394
395
396
397
398
399
400
401
402
403
404
405
406
407
408
409
410
411
412
413
414
415
416
417
418
419
420
421
422
423
424
425
426
427
428
429
430
431
432
433
434
435
436
437
438
439
440
441
442
443
444
445
446
447
448
449
450
451
452
453
454
455
456
457
458
459
460
461
462
463
464
465
466
467
468
469
470
471
472
473
474
475
476
477
478
479
480
481
482
483
484
485
486
487
488
489
490
491
492
493
494
495
496
497
498
499
500
501
502
503
504
505
506
507
508
509
510
511
512
513
514
515
516
517
518
519
520
521
522
523
524
---
title: "Bayes"
author: "Dominique Gravel & Andrew MacDonald"
date: "August 26th, 2021"
output:
xaringan::moon_reader:
css: [default, assets/ecl707.css, "hygge"]
lib_dir: assets
seal: false
nature:
highlightStyle: monokai
highlightLines: true
countIncrementalSlides: false
beforeInit: "macros.js"
---
class: title-slide, middle
<style type="text/css">
.title-slide {
background-image: url('assets/img/bg.jpg');
background-color: #23373B;
background-size: contain;
border: 0px;
background-position: 600px 0;
line-height: 1;
}
</style>
# Model evaluation with ecological data
<hr width="65%" align="left" size="0.3" color="orange"></hr>
## Bayesian approaches
<hr width="65%" align="left" size="0.3" color="orange" style="margin-bottom:40px;" alt="@Martin Sanchez"></hr>
.instructors[
**ECL707/807** - Dominique Gravel
]
<img src="assets/img/logo.png" width="25%" style="margin-top:20px;"></img>
---
# Bayesian Philosophy
What makes a Bayesian way of thinking?
--
$$
P(A|B) = \frac{P(B|A)P(A)}{P(B)}
$$
is it Bayes Rule?
--
Not entirely! On it's own, this Rule is just logic/algebra
---
# Bayesian Philosophy
Bayesians quantify uncertainty with probability
```{r echo=FALSE, message=FALSE, warning=FALSE}
suppressPackageStartupMessages(library(tidyverse))
betacurve <- function(x, phi) dbeta(x, .4*phi, (1-.4)*phi)
tibble(x = seq(from = 0.01, to = 0.99, length.out = 100),
y1 = betacurve(x, 4),
y2 = betacurve(x, 10),
y3 = betacurve(x, 20)) %>%
pivot_longer(-x) %>%
ggplot(aes(x = x, y = value, colour = name)) +
guides(color = FALSE) +
geom_line(lwd = 3) +
theme_bw()+
scale_color_brewer(type = "qual") +
labs(x = "Probability of Survival",
y = "Density")
```
---
# Bayesian Philosophy
In other words: Bayesians consider that the scientific world is divided into two parts:
* .huge[**The Observed**]: Data! the observations, numbers, photos, "y variables" whatever you call them
--
* .huge[**The Unobserved**]: parameters, latent states, usually of much more general scientific interest
---
class: center
# Bayesian Philosophy
How do we use the observed to learn about the unobserved?
We use:
$$
[y, \theta]
$$
the **joint distribution of the parameters and the data**
---
uh what kind of joint is this
.pull-left[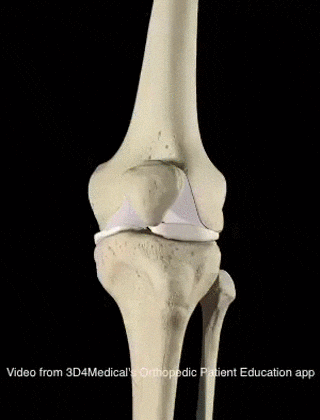]
.pull-right[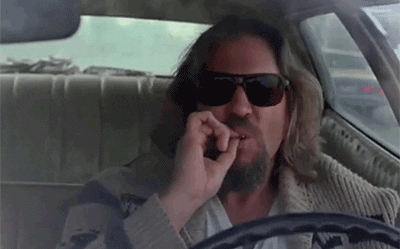]
---
# Bayesian Philosophy
.pull-left[A simple sketch of a joint distribution: when a parameter changes (survivorship probability) the observations also change. Likewise, we might think that a change in the observations suggests that the unseen probability has also changed.]
.pull-right[
```{r echo = FALSE}
tibble(p = rep(c(.2, .6, .9), each = 10),
survived = rbinom(30, 10, prob = p)) %>%
ggplot(aes(x = p, y = survived)) + geom_point(position = position_jitter(width = .05), size = 3) +
theme_bw() +
labs(x = "Probability of survivorship", y = "Survival")
```
]
---
# Bayesian Philosophy
We _factor_ the joint distribution into two parts, like this:
$$
[y, \theta] = [y | \theta]\times [\theta]
$$
--
* $[y|\theta]$ is the Likelihood
--
* $[\theta]$ is the prior
--
And finally apply Bayes Rule
$$
[\theta|y] = \frac{[y|\theta][\theta]}{[y]}
$$
This numerator involves summing up the likelihood over **all possible prior values**. In other words, considering what DID happen relative to everything that _could have_ happened!
---
# Goal for today
Today we will build intuition for Bayesian inference. By the end of today (or tomorrow!) you will have learned how to find, and work with, the posterior distributions of parameters
We'll look at the following topics
* Posterior via grid search
--
* Posterior via conjugate priors
--
* Metropolis and Metropolis-Hastings algorithms
--
_brief detour into Hierarchical Models!_
--
* Gibbs sampling
---
class: centre
# Grid Search
*Objective* intuition for how we actually multiply the prior by the likelihood to get a probability density function.
Grid search is very similar to our initial Maximum Likelihood procedures, with the addition of a prior
---
# Chick survival
The Fake Petrel ( _Hydrobates inventus_ ) lays precisely 6 eggs. You have data on survivorship from 10 different nests:
```{r echo = TRUE}
set.seed(1859)
x_obs <- rbinom(10, 6, 0.6)
x_obs
```
Our model is:
$$
\text{hatched}_{i} \sim \text{Binomial}(\theta, 6)
$$
Because we want the Bayesian posterior distribution, we also need a prior for $\theta$
$$
\theta \sim \text{Uniform}(0,1)
$$
We'll try several, but this is our starting point
---
Use grid search to find the posterior distribution of $\theta$, the probability that an egg successfully hatches.
# Steps
1. make a vector of probabilities for $\theta$ using `seq(from = 0, to = 1, length.out = n)`
1. Think about the prior you might use. Start with `dunif(x, min = 0, max = 1)`.
1. find the probability of each of these values of $\theta$ via `dunif`
1. find the likelihood for each of these values of $\theta$ via `dbinom`
1. multiply these two columns together
1. normalize (divide by the sum)
---
# Notes and reminders
A note on the Beta distribution:
$$
\text{Beta}(\alpha = \mu\phi, \beta = (1 - \mu)\phi)
$$
* $\mu$ the average probability
* $\phi$ the "concentration" around this value
---
class: center
# conjugate priors

---
# Conjugate priors
Conjugate priors exist for many common probability distributions
The posterior is the same distribution as the prior with new, updated parameters
--
> it is embarassing to do an elaborate numerical procedure to obtain results that can be obtained on a napkin -- Hobbs and Hooten
--

---
$$[\theta] = {\theta^{\alpha-1}(1-\theta)^{\beta-1} \over \text{Beta}(\alpha,\beta)}$$
$$[y|\theta] = {n \choose y}\theta^y(1-\theta)^{n-y}$$
$$
[y|\theta][\theta] \propto \theta^{\alpha-1}(1-\theta)^{\beta-1} \times \theta^y(1-\theta)^{n-y}
$$
$$
[y|\theta][\theta] \propto \theta^{y + \alpha-1}(1-\theta)^{n - y + \beta-1}
$$
$$
[\theta|y] = \frac{\theta^{y + \alpha-1}(1-\theta)^{n - y + \beta-1}}{ \text{Beta}(\alpha + y,\beta + n-y)}
$$
---
# Conjugate priors
| prior | likelihood | Posterior |
|-------------------|-----------------------|------------------------------------------------------------------|
| $p ~\sim \text{Beta}(\alpha,\beta)$ | $y \sim \text{Binomial(p, N)}$ | $p \sim \text{Beta}(\alpha + \sum y, \beta + \sum (n- y)$ |
| $\lambda \sim \text{gamma}(\alpha, \beta)$ | $y \sim \text{Poisson}(\lambda)$ | $\lambda \sim \text{gamma}(\alpha + \sum y, \beta + n)$ |
---
# Petrels again
Lets go back to our Fake Petrel data
```{r echo = TRUE}
set.seed(1859)
x_obs <- rbinom(10, 6, 0.6)
x_obs
```
Calculate the conjugate posterior and add it to the figure using `curve`. How does it compare to grid search.
---
# Predictions
In both of these approaches, we end up with a _distribution_ for p. How can we use this to make predictions?
* sample values from your posterior for $p$
* for each sampled value, sample a single surviving nest of 6 eggs
* do this lots of times
_remember R is vectorized_
--
e.g. for prior $\mu = 0.3$ and $\phi = 5$
```{r }
ps <- rbeta(200, 0.3 * 5 + sum(x_obs),
0.7 * 5 + sum(6 - x_obs))
ys <- rbinom(200, size = 6, prob = ps)
```
---
# Exercise with your data
does the Distribution you chose have a conjugate prior? Can you estimate the posterior distribution this way?
---
class: inverse, centre
# Markov Chain Monte Carlo
---
# Remember Simulated Annealing
Your grasp of simulated annealing will extend to understanding MCMC methods as well!
Three things to keep in mind:
* We can work with a function even if it is too complex to analyze directly
--
* the *Effeciency* of an algorithm is key, especially when problems grow
--
* We get several knobs to turn to control how an algorithm behaves. Another thing to think about as you perform modelling!
---
# MCMC "magic"
The objective of MCMC algorithm is to simulate parameters from a complex posterior distribution.
--
Rather than measure the *absolute* probability of different parameter values:
$$
[\theta|y] = \frac{[y|\theta][\theta]}{[y]}
$$
we sample, and evaluate the *relative* probability of two possible parameter values:
$$
\frac{[\theta^{new}|y]}{[\theta^k|y]} = \frac{[y|\theta^{new}][\theta^{new}]}{[y|\theta^k][\theta^k]}
$$
In this way, we can sample a probability distribution ( $[\theta|y]$ ) using only something _proportional_ to that distribution ( $[y|\theta][\theta]$ )
---
# The Metropolis Algorithm
* Draw $\theta^{new}$ from a _symmetric_ distribution centered on $\theta^{k}$ (i.e. in the $k$th interation, the current value)
e.g. $\theta^{new} \sim N(\theta^{k}, \sigma_{tune})$
* Calculate the ratio R:
$$
R = \text{min}\left( 1, \frac{[y|\theta^{new}][\theta^{new}]}{[y|\theta^k][\theta^k]}\right)
$$
This is the probability of acceptance. In other words, if the ratio is better always take it. If the ratio is less than 1, there's a chance we'll take it anyway.
* Test to see if `runif(1, min=0, max=1)` $< R$. if so, set $\theta^{k + 1} = \theta^{new}$
* **Discussion question**: why should we accept "worse" parameter values? Why is it good to sometimes stay in the same place?
---
class: center
# Visualizing
```{r echo = FALSE, fig.retina=3}
suppressPackageStartupMessages(library(tidyverse))
tibble(x = seq(0, 10, length.out = 200),
y = dnorm(x, mean = 5, sd = 2)) %>%
ggplot(aes(x, y)) + geom_line() +
theme_bw() +
theme(axis.text = element_blank())+
geom_vline(xintercept = 5, lty = 2) +
labs(y = NULL, x = "Old value") +
annotate("segment", x = 5.1, xend = 7, y = .1, yend = .1, arrow = arrow()) +
annotate("segment", x = 4.9, xend = 3, y = .1, yend = .1, arrow = arrow()) +
annotate("text", x = 6, y = .11, label = "sigma[tune]", parse = TRUE)
```
---
class: center
# Visualizing
```{r echo = FALSE, fig.retina=3}
tibble(x = seq(0, 10, length.out = 200),
y = dnorm(x, mean = 5, sd = 2)) %>%
ggplot(aes(x, y)) + geom_line() +
theme_bw() +
theme(axis.text = element_blank())+
geom_vline(xintercept = 5, lty = 2) +
labs(y = NULL, x = "Old value") +
geom_vline(xintercept = 6.7, lty = 2) +
annotate("text", y = 0.01, x = 7.5, label = "new value??")
```
---
# quick reminder!
--
* the candidate distribution is not the prior
--
* the candidate distribution is not the prior
--
* the candidate distribution makes new values but it's not the prior, you still have to put the values it produces into the likelihood and the prior
---
# Now: to math!
$$
r = \frac{\text{Likelihood}(\text{data}|\theta^{new}) \times \text{Prior}(\theta^{new}, \alpha, \beta)}{\text{Likelihood}(\text{data}|\theta^{old}) \times \text{Prior}(\theta^{old}, \alpha, \beta)}
$$
* here I'm using $\alpha$ and $\beta$ to mean any prior parameters. They don't change!
* plug the new candidate value into the likelihood and multiply by the prior
---
# Try it out!
Use the Metropolis algorithm to fit the following data on the Fictitious Leafhopper abundance on 5 plants:
```{r echo=TRUE}
set.seed(1859)
xs <- rpois(5, 27)
xs
```
$$
\begin{align}
y &\sim \text{Poisson}(e^{\alpha}) \\
\alpha &\sim \text{Normal}(10, 2)
\end{align}
$$
_suggestion_ use as a candidate distribution $\text{Normal}(\theta^k, \sigma_{tune})$
This means making your guesses with `rnorm(1, mean = old_param, sd = tune)`
**Compare your result to the conjugate posterior! you should get very similar shapes**
---
# Metropolis Hastings
Sometimes you need an _asymmetrical_ distribution for your parameter. This happens when the parameter is bounded.
In this case, we need to adjust our calculation of $r$. Now in addition to the Likelihood and Prior, we have the Candidate distribution getting involved
$$
\frac{[y|\theta^{new}][\theta^{new}][\theta^{old}|\theta^{new}]}
{[y|\theta^{old}][\theta^{old}][\theta^{old}|\theta^{new}]}
$$
.small[
<p>
$$
r = \frac{\text{Likelihood}(\text{data}|\theta^{new}) \times \text{Prior}(\theta^{new}, \alpha, \beta) \times \text{Candidate}(\theta^{old}, \mu = \theta^{new}, \sigma_{tune})}{\text{Likelihood}(\text{data}|\theta^{old}) \times \text{Prior}(\theta^{old}, \alpha, \beta)\times \text{Candidate}(\theta^{new}, \mu = \theta^{old}, \sigma_{tune})}
$$
</p>
]
then finally
$$
R = \text{min}( 1, r)
$$
---
# Try it out!
Use the Metropolis-Hastings algorithm to fit the following data on the Fictitious Leafhopper abundance on 5 plants:
```{r echo=TRUE}
set.seed(1859)
xs <- rpois(5, 27)
xs
```
$$
\begin{align}
y &\sim \text{Poisson}(\lambda) \\
\lambda &\sim \text{Gamma}\left(\frac{25^2}{15^2}, \frac{25}{15^2}\right)
\end{align}
$$
_suggestion_ use as a candidate distribution $\text{LogNormal}(log(\theta^k), \sigma_{tune})$
This means making your guesses with `rlnorm(1, mean = log(old_param), sd = tune)`
**Compare your result to the conjugate posterior! you should get very similar shapes**
---